Chapitre 4 Profiler son code
On parle de profiling en anglais. Il sâagit de dĂ©terminer ce qui prend du temps dans un code. Le but Ă©tant, une fois trouvĂ© le bloc de code qui prend le plus de temps dans lâexĂ©cution, dâoptimiser uniquement cette brique.
Pour obtenir un profiling du code ci-dessous, sélectionner les lignes de code
dâintĂ©rĂȘt et aller dans le menu âProfileâ puis âProfile Selected Linesâ. Cela utilise en fait la fonction profvis()
du package profvis
.
n <- 10e4
pdfval <- mvnpdf(x=matrix(1.96, nrow = 2, ncol = n), Log=FALSE)
OK, we get it ! ConcatĂ©ner un vecteur au fur et Ă mesure dans une boucle nâest vraiment pas une bonne idĂ©e.
4.1 Comparaison avec une version plus habile de mnvpdf()
Considérons une nouvelle version de mvnpdf()
, appelée mvnpdfsmart()
. TĂ©lĂ©charger le fichier puis lâinclure dans votre package.
Profiler la commande suivante :
n <- 10e4
pdfval <- mvnpdfsmart(x=matrix(1.96, nrow = 2, ncol = n), Log=FALSE)
On a effectivement résolu le problÚme et on apprend maintenant de maniÚre plus fine ce qui prend du temps dans notre fonction.
Pour confirmer que mvnpdfsmart()
est effectivement bien plus rapide que
mvnpdf()
on peut re-faire une comparaison avec microbenchmark()
:
n <- 1000
mb <- microbenchmark(mvnpdf(x=matrix(1.96, nrow = 2, ncol = n), Log=FALSE),
mvnpdfsmart(x=matrix(1.96, nrow = 2, ncol = n), Log=FALSE),
times=100L)
mb
## Unit: milliseconds
## expr min
## mvnpdf(x = matrix(1.96, nrow = 2, ncol = n), Log = FALSE) 3.138591
## mvnpdfsmart(x = matrix(1.96, nrow = 2, ncol = n), Log = FALSE) 2.330481
## lq mean median uq max neval cld
## 3.322681 3.778820 3.437030 3.580571 8.138008 100 a
## 2.360309 2.447065 2.381362 2.425847 6.387636 100 b
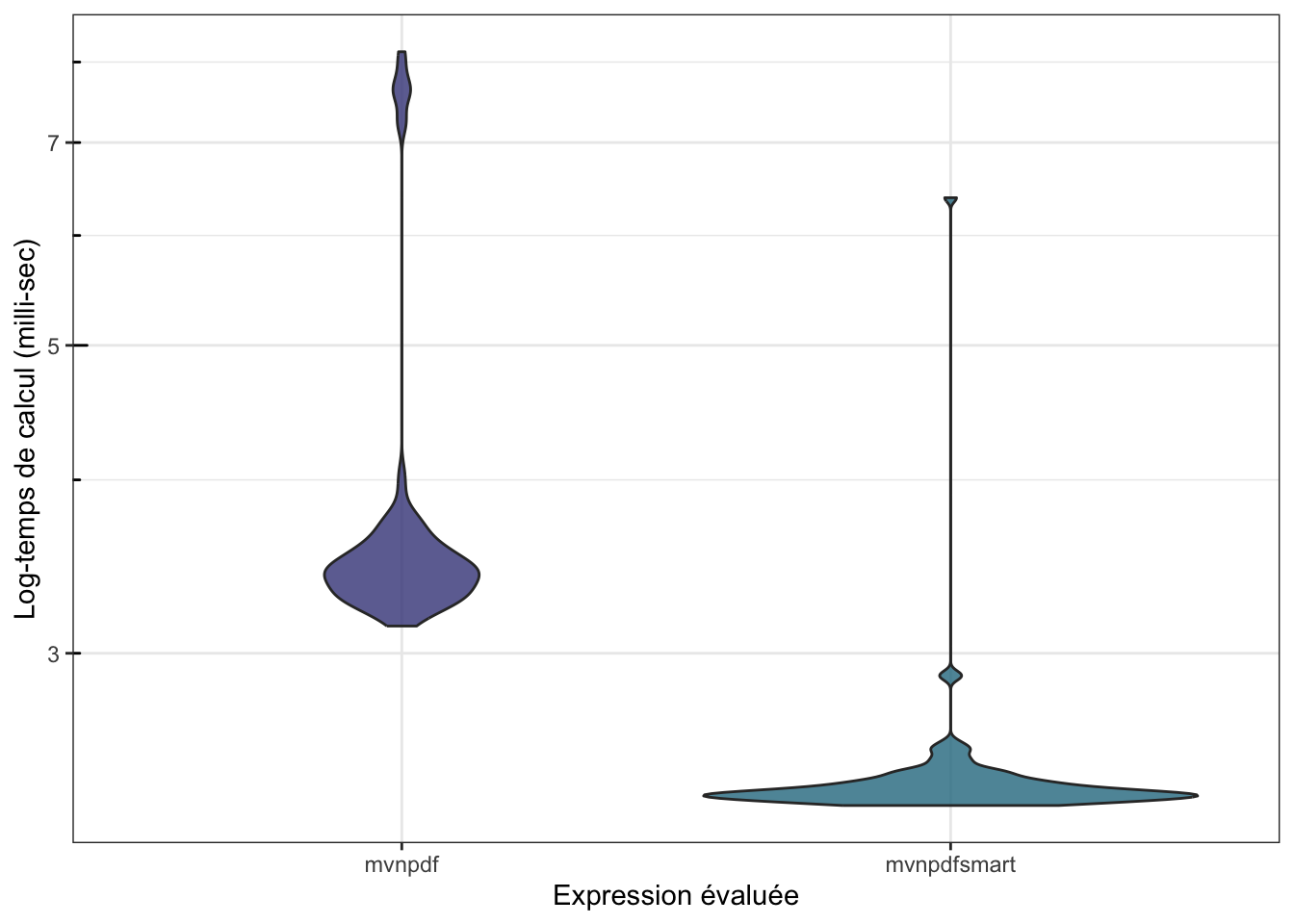
Et on peut également voir si on devient compétitif avec dmvnorm()
:
n <- 1000
mb <- microbenchmark(mvtnorm::dmvnorm(matrix(1.96, nrow = n, ncol = 2)),
mvnpdf(x=matrix(1.96, nrow = 2, ncol = n), Log=FALSE),
mvnpdfsmart(x=matrix(1.96, nrow = 2, ncol = n), Log=FALSE),
times=100L)
mb
## Unit: microseconds
## expr min
## mvtnorm::dmvnorm(matrix(1.96, nrow = n, ncol = 2)) 43.747
## mvnpdf(x = matrix(1.96, nrow = 2, ncol = n), Log = FALSE) 3147.857
## mvnpdfsmart(x = matrix(1.96, nrow = 2, ncol = n), Log = FALSE) 2308.546
## lq mean median uq max neval cld
## 51.8445 73.05421 74.251 89.749 117.424 100 a
## 3295.1085 3715.51266 3416.387 3522.740 8054.737 100 b
## 2348.0085 2504.66540 2379.497 2428.163 10087.189 100 c
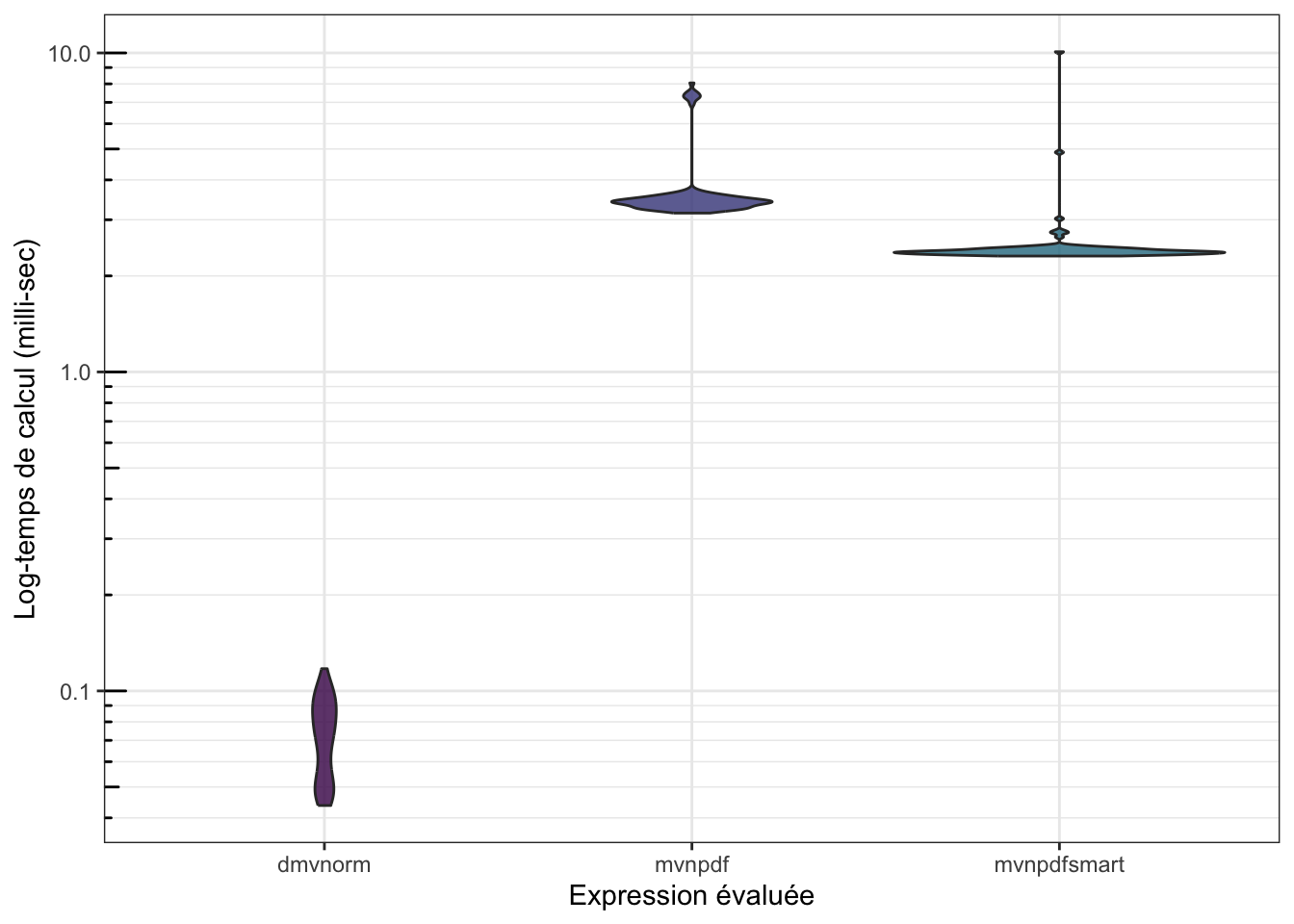
Il y a encore du travailâŠ
4.2 Comparaison avec une version optimisée dans
Boris est arrivé, aprÚs de longues recherches et plusieurs tests, à une version optimisée avec les outils de .
Inclure la fonction mvnpdfoptim()
dans le package, puis profiler cette
fonction :
Et un petit microbenchmark()
:
n <- 1000
mb <- microbenchmark(mvtnorm::dmvnorm(matrix(1.96, nrow = n, ncol = 2)),
mvnpdf(x=matrix(1.96, nrow = 2, ncol = n), Log=FALSE),
mvnpdfsmart(x=matrix(1.96, nrow = 2, ncol = n), Log=FALSE),
mvnpdfoptim(x=matrix(1.96, nrow = 2, ncol = n), Log=FALSE),
times=100L)
mb
## Unit: microseconds
## expr min
## mvtnorm::dmvnorm(matrix(1.96, nrow = n, ncol = 2)) 43.337
## mvnpdf(x = matrix(1.96, nrow = 2, ncol = n), Log = FALSE) 3154.499
## mvnpdfsmart(x = matrix(1.96, nrow = 2, ncol = n), Log = FALSE) 2314.450
## mvnpdfoptim(x = matrix(1.96, nrow = 2, ncol = n), Log = FALSE) 1747.871
## lq mean median uq max neval cld
## 53.6075 73.60197 75.235 90.3025 142.680 100 a
## 3301.1970 3824.36479 3398.121 3571.4485 8685.276 100 b
## 2368.7135 2419.81877 2401.309 2434.4365 3397.875 100 c
## 1794.3855 1922.38012 1828.580 1863.3475 6635.522 100 d
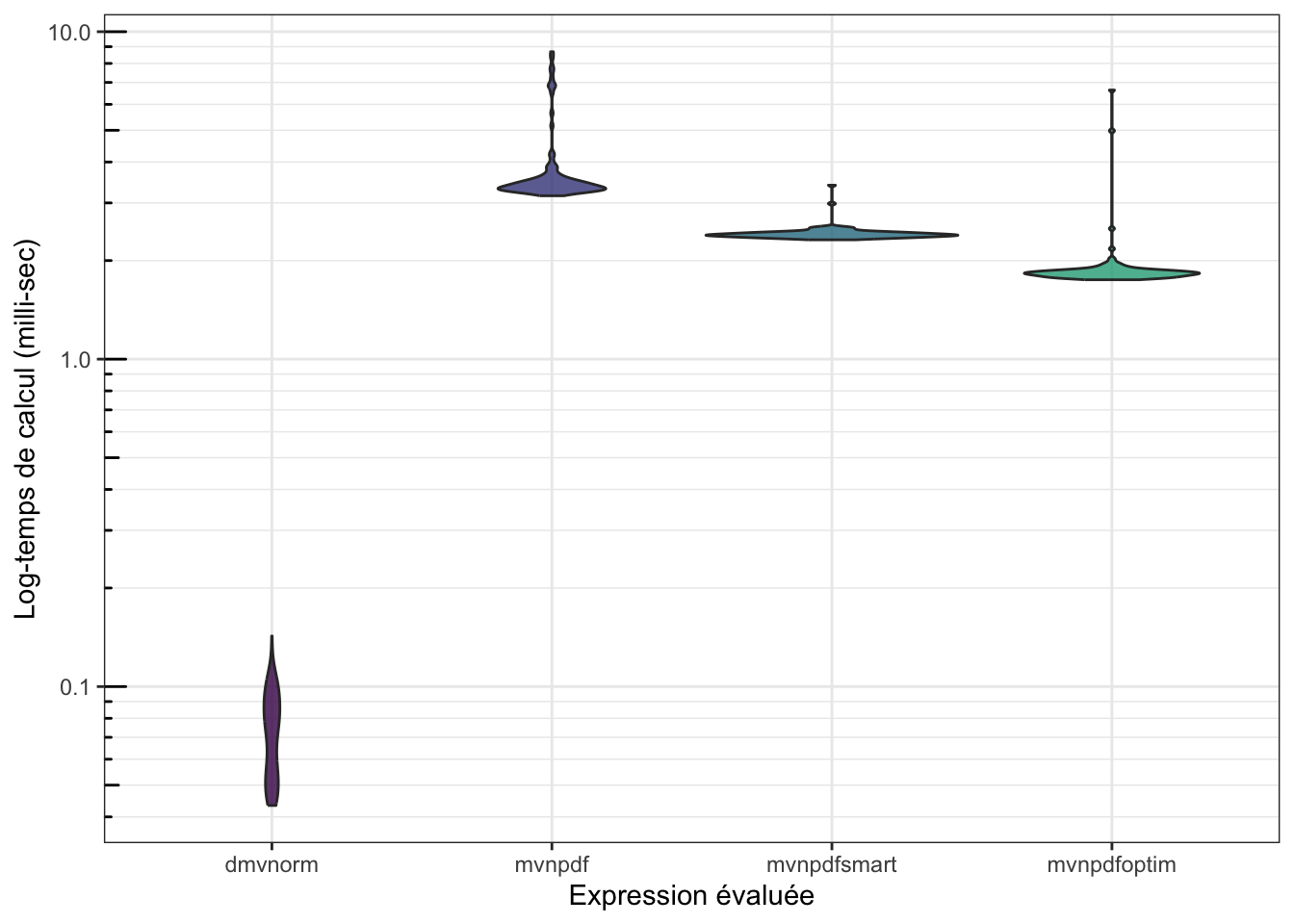
Pour finir on peut profiler la fonction dmvnorm()
: